Navigating the AI Adoption Ladder
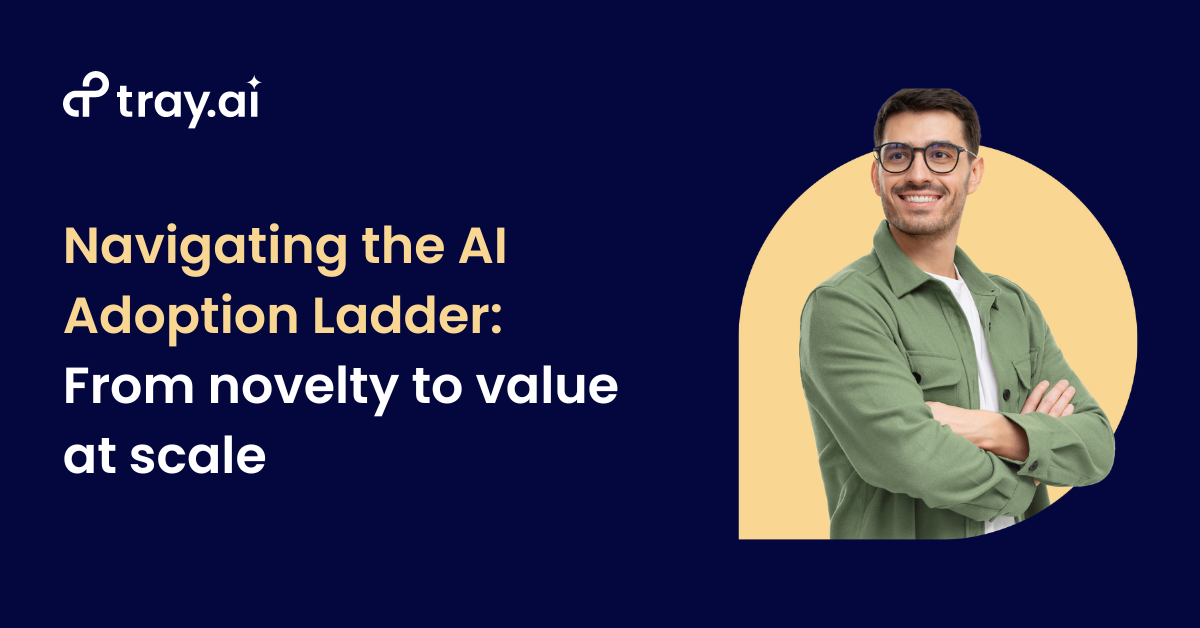
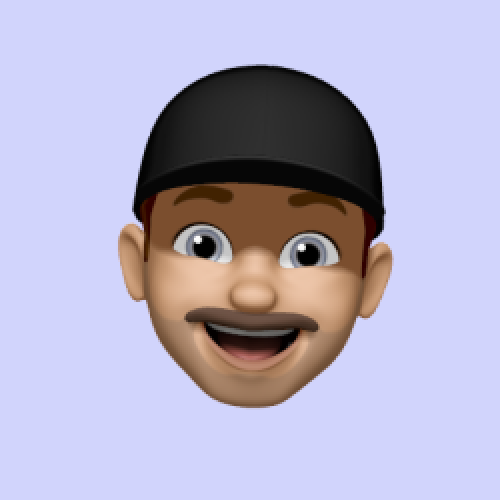
Austin Johnson
Explore the AI Adoption Ladder: a guide to transitioning from AI curiosity to strategic integration, unlocking transformative growth and competitive edge.
(This post was originally published in December 2023, updated in November 2024)
Hey, I'm Austin, Product Manager at Tray.ai. AI is changing the ways we work. At Tray we have Native AI Capabilities, 3rd party AI connectors, templates, and frameworks that you can use today to get started.
This blog is all about the first steps on your journey to unlocking genuine business impact with AI. If you are well on your way we have other blogs that are likely a better fit for you (read up on knowledge agents in more detail here).
What we cover:
The AI Adoption Ladder
Key AI terms / concepts
Advice on how to run your first AI tests
First, a quick reflection on how we got here…
Think back a year ago.
Many folks first interacted with AI tools like Anthropic's Claude or OpenAI's ChatGPT, marveling at their novelty and potential.
We asked simple questions and received instant, human-like responses, igniting a realization that AI was more than just a fleeting trend – it was going to revolutionize how we work.
Now think about the last few months - you were still excited, but you felt stuck. You are trying to get real value and help from AI to do your job, but it’s falling short.
Sound familiar?
Fast forward to today, and the landscape has shifted dramatically. Companies are now actively integrating AI into their process and tool stacks.
Leadership at most companies says the impact on your business needs to be 10x.
This year the leading businesses in your industry were experimenting, testing, and deploying AI across all processes.
New research by Tray.ai reveals that 9 out of 10 enterprises face challenges integrating AI into their tech stack.
It isn't too late to start working your way up the AI Adoption Ladder.
To achieve this transformation, you and your team (and your org) need to develop competencies in AI and APIs. This involves embracing three core principles: developing skills, owning data, and staying nimble. We will come back to these.
First, a bit more about the AI Adoption Ladder…
The AI Adoption Ladder explained
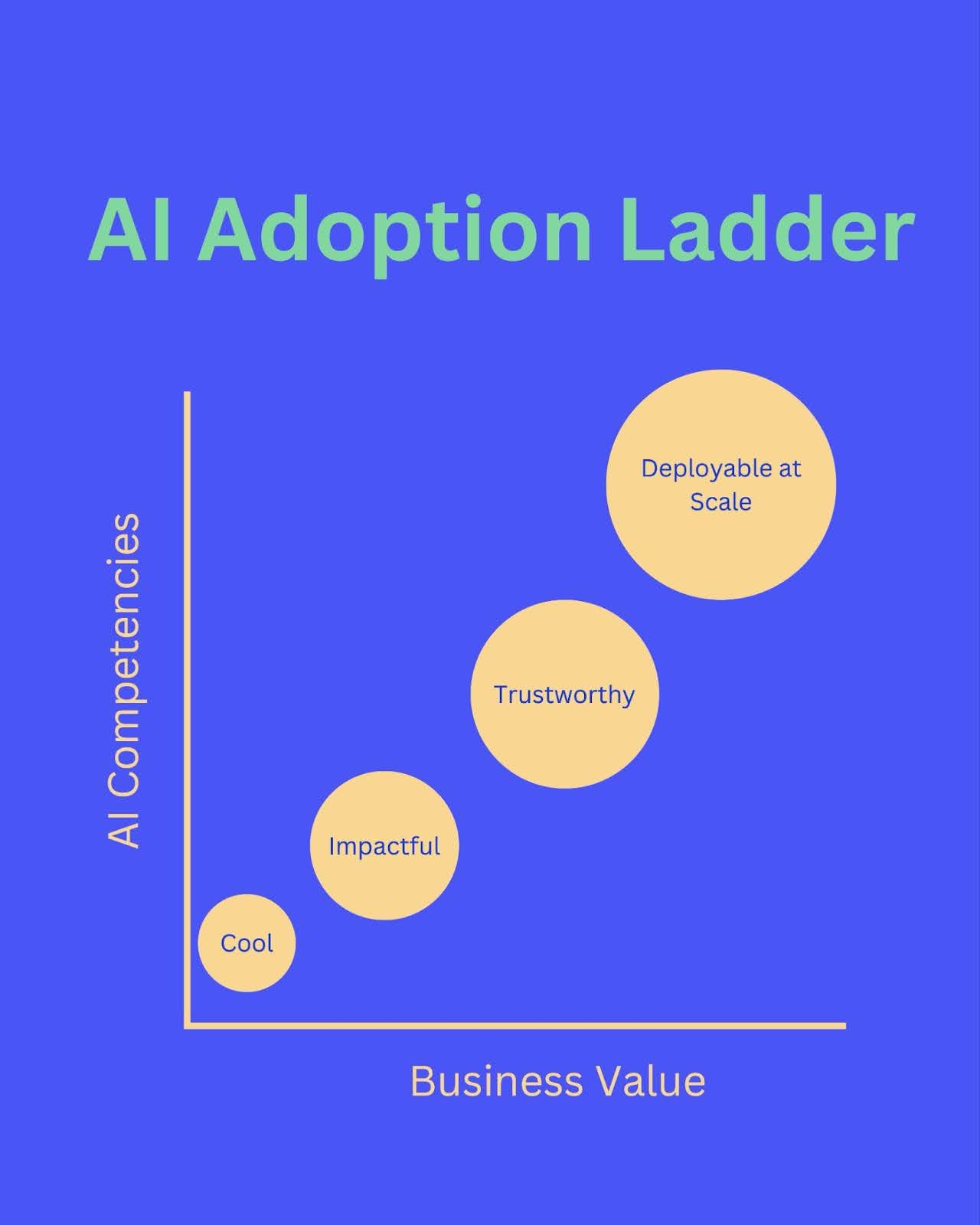
The AI Adoption Ladder illustrates the progression from perceiving AI as a cool toolset to fully integrating it as a significant driver of organizational value.
As you build AI competencies, you and your team can drive more business value. Each level of value has specific competencies that you can develop in your team.
Stage 1: Cool and novel use of AI
Stage 2: Impactful - value is happening in silos and for individuals
Stage 3: Trustworthy - AI can be used across departments and responses provide citations because you are grounding it with your own proprietary data
Stage 4: Deployable at Scale - you are running production-level tooling and driving significant business value with AI
Now that you know the path, let’s talk about the principles that will help get you there…
The three core principles for AI adoption
Develop the Skills: Skill development is key to AI adoption. Cultivating an environment of experimentation and API literacy is vital, not just for individuals but for teams as well.
Own the Data: Your proprietary data is your asset. Relying solely on common tools provides no competitive edge. Your unique application of AI to your data is where true value lies.
Stay Nimble: The AI landscape is perpetually evolving. Building flexible, adaptable processes allows you to swiftly incorporate new developments and maintain a competitive edge.
These principles are universal and will hold steady through the fast pace of change. Let’s cover some of the landscape as it sits today…
Vendor landscape and key concepts
The AI vendor landscape is dynamic, featuring major players like AWS, Microsoft, and Google. Understanding key concepts such as Large Language Models (LLMs), embedding, and vector storage is crucial. This knowledge helps in navigating the complex relationships between vendors and AI functionalities.
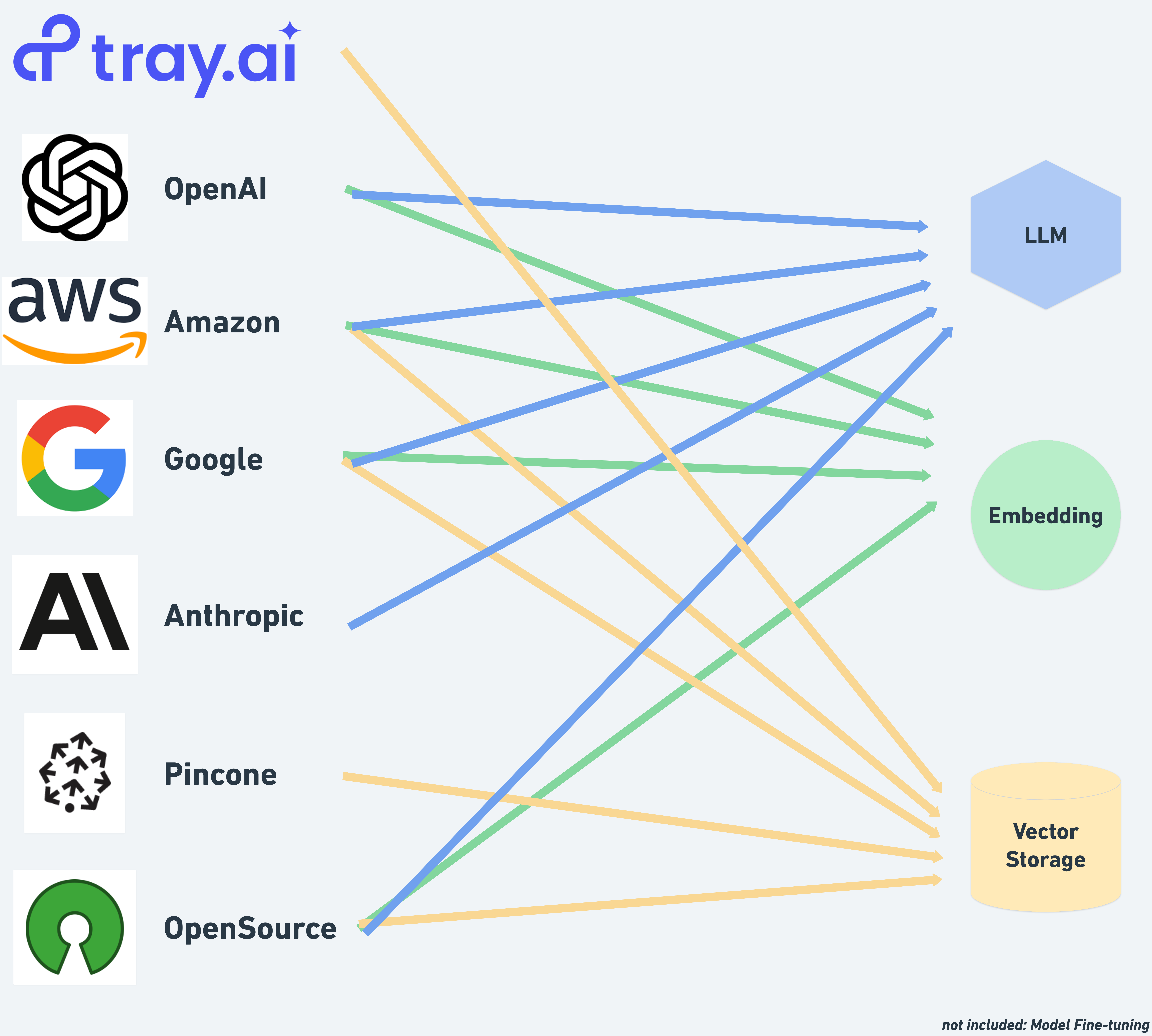
Large Language Models (LLMs) like GPT-4o by OpenAI or Claude 3.5 Sonnet by Anthropic are at the forefront of AI technology, allowing machines to understand and generate human-like text.
Embedding is a way to simplify complex information into a format that AI systems can understand and use quickly, similar to converting diverse text data into a universal language of numbers. Think of it as creating a digital map, where each piece of data is assigned its own semantic 'location' for easy access.
Vector storage acts like an efficient filing system for this 'digital map,' ensuring that the information is organized in such a way that it can be accessed, analyzed, and utilized swiftly by AI processes, systems, and apps. It is a core part of most production Retrieval Augmented Generation pipelines.
As for the big AI players out there today, vendors such as AWS’s Bedrock, Microsoft’s Azure AI, and Google’s Vertex AI Platform all offer unique features and capabilities. For example, Bedrock helps you quickly build, train, and deploy learning models, Azure AI includes various AI services, and Google’s Vertex AI Platform allows for large-scale AI model deployment.
While these are just a few of the big innovators in AI, it’s important to remember that the vendor landscape is changing constantly.
Do not get bogged down by this knowledge or memorize it. Remember, if you develop the skills you will advance alongside the technology.
Make sure that your frameworks and experiments are built to stay nimble and you will not have to be a master of the tool landscape. You know you can quickly switch and experiment and won’t fall behind as developments are made.
It is helpful to go beyond words here. Let’s talk about a concrete use-case that you can experiment with…
Get started experimenting: Conversational AI architecture
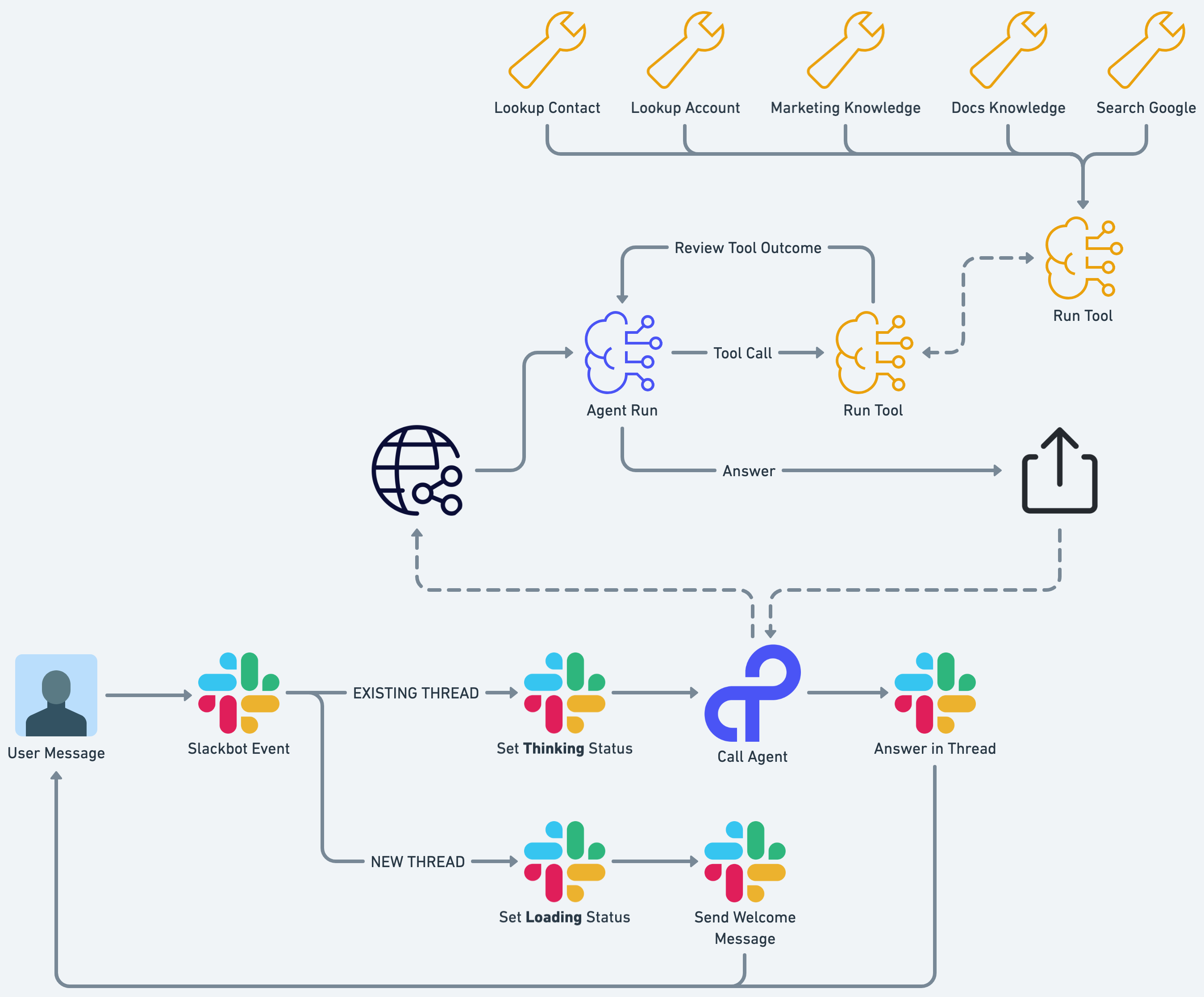
We developed this introductory architecture to help you develop the skills. This architecture mimics the functionality of ChatGPT (having a conversation with a generalized AI), but allows you to have control of the prompt engineering layer AND distribute it to ANY user interface.
Here's an explanation of the different components and how they interact with each other:
Slack: This is where the user inputs their query or prompt. It's the front-end part of the system that interacts directly with the user. Internally we use Slack (as one of the interfaces), but this can be any application where you can chat via APIs (ie MS Teams).
Thread/New Request: The system determines if the input is a net new query, or the continuation of a conversation and handles it within that context.
Agent Layer: Here, we use an Agent to ground the response in the knowledge of our business data using multiple potential data sources. It can reason (identify which tools or knowledge to tap into), act (actually interact with external tools), and answer using all this background work.
Respond to the User: You surface the response to the user at the same (or any additional) user interface so they can continue chatting.
You can update or swap out any of the components with your tools of choice. This system not only provides real-time responses but also adapts to the conversational context, enhancing the user experience.
Next up: Trustworthiness and beyond
The AI Adoption Ladder is more than just a concept; it's a roadmap for transforming your business with AI. The next step is to build trustworthiness in AI applications. This involves delving deeper into AI functionalities and ensuring that AI-driven solutions are reliable and robust.
Want help getting to the next rung on the AI Adoption Ladder?